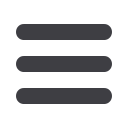
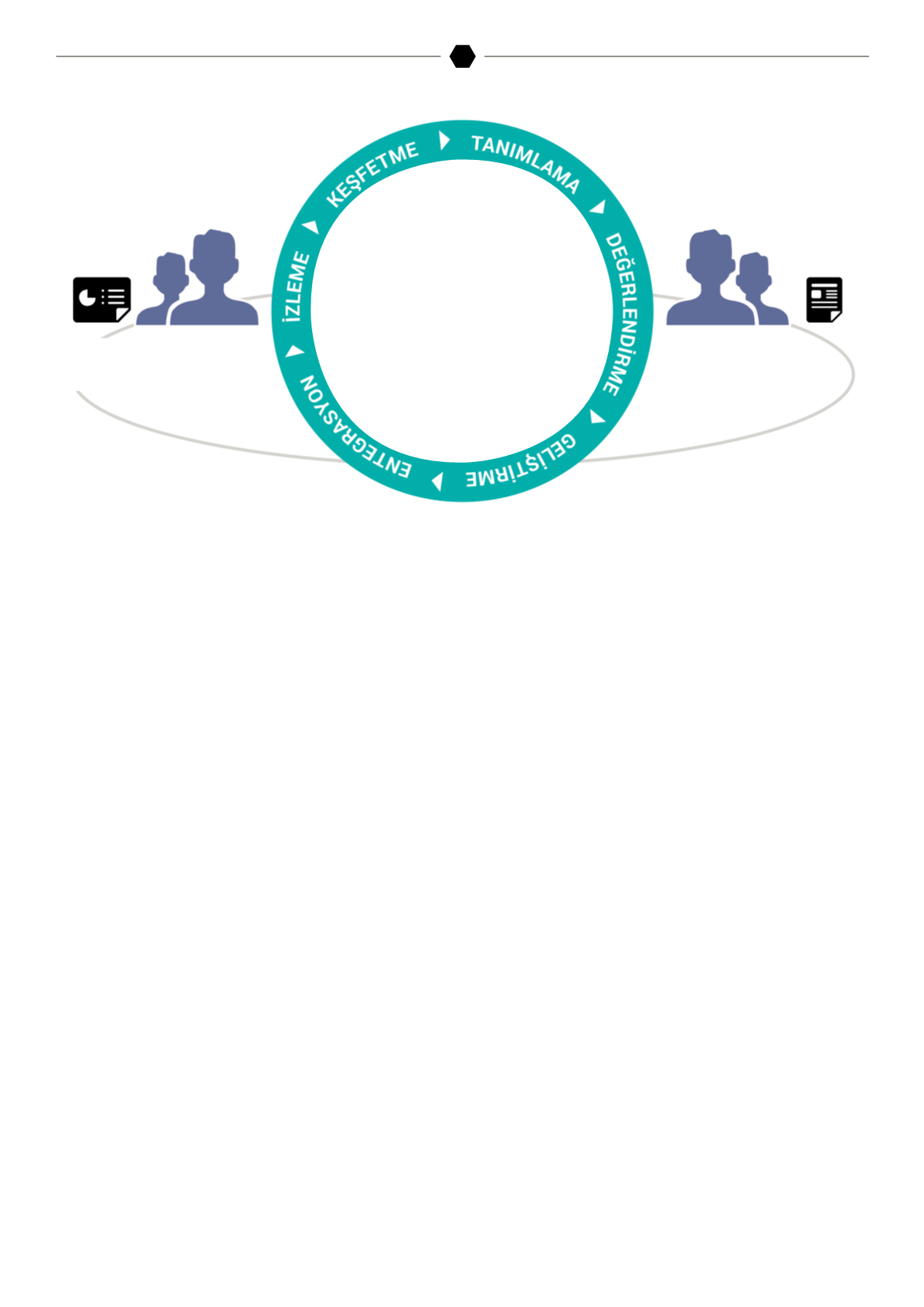
59
Veri kalitesi, yaşayan bir organizasyon olarak ele
alınmalı ve “insan –teknoloji – süreç” üçgeninde
değerlendirilmelidir.
Gartner araştırma sonuçlarına göre;
İş dünyasının çok büyük bir kısmı data kalitesi
süreçlerini otomatize edememiştir.
Kurumların işletme gelirlerinin yüzde 10-20’si
veri kalitesi ile ilişkili problemlerin giderilmesi için
harcanmaktadır.
Veri ambarı projelerinin yüzde 50’sinden fazlası veri
kalitesindeki problemleri giderememe nedeniyle
başarısızlığa uğramaktadır.
Birçok kurum gerçek veri yönetimi prensiplerini
anlamamaktadır. Veri kalitesi sadece veri temizleme
anlamına gelmemektedir.
Bu araştırma bir kurumda veri kalitesi süreçlerini
oturtmanın çok kolay bir iş olmadığını ve bu süreçlerin
işlerlik kazanmaması da yüksek maliyetlere neden
olduğunu ortaya koymaktadır.
Veri kalitesi
çalışmalarında
başarı
sağlayabilmek için
şu
adımların
takip edilmesi faydalı olacaktır:
Ölçülebilir hedefler belirlenmelidir
Başarının en önemli faktörlerinden biri projeden
beklenen iş faydalarını tanımlamaktır. Fatura
maliyetlerini düşürmek için müşteri listelerini konsolide
etmek, aynı müşteriye ait farklı departmanlardaki
verileri gözden geçirmek ve suistimal tespiti
yapabilmek için müşterileri tanımlamak gibi spesifik
Data quality should be viewed as a living organization
and should be evaluated within the triangle of
“man-technology-process”.
According to Results of Research by Gartner;
A large portion of the business world has not yet
automated their data quality processes.
Around 10 to 20% of the income of the corporations is
spent on developing solutions to problems related to
data quality.
More than 50% of the data warehousing projects fail
due to failure to solve problems related to data quality
Most organizations fail to grasp the real data
management principles. Data quality is not only about
cleaning up data.
This study shows that establishing data quality processes
in an organization is not an easy task and that failure to
activate such processes results in high costs.
For efforts aimed at improving data quality to be
successful, it would be helpful to follow the below
mentioned steps:
Measurable goals should be determined
One of the most important elements of success is
defining the expected business benefits of a project.
Specific goals such as consolidating customer lists
to decrease invoicing expenses, to review information
from different departments related to the same
customer and to define customers in order to detect
fraud are crucial for the success of a project. These
goals need to be measurable at the same time. A solid
goal such as decreasing multiple customer data by
UZMAN GÖZÜYLE
EXPERT VIEW
Kurallarin Geliştirilmesi
Creating Rules
Profilleme
Profiling
Temizlik & Entegrasyon
Clean up and Integration
Bilgi Teknolojileri Geli;tiricileri
Information Technology Developers
İş Birimi
Business unit
VERİ KALITESİ KURALLARI
Rules for Data Quality
Kurallarin Tanimlanmasi
Defining Rules
Sozluk ve Referans
Glossary and Reference Sources
Listelerin yönetilmesi
Managing the Lists